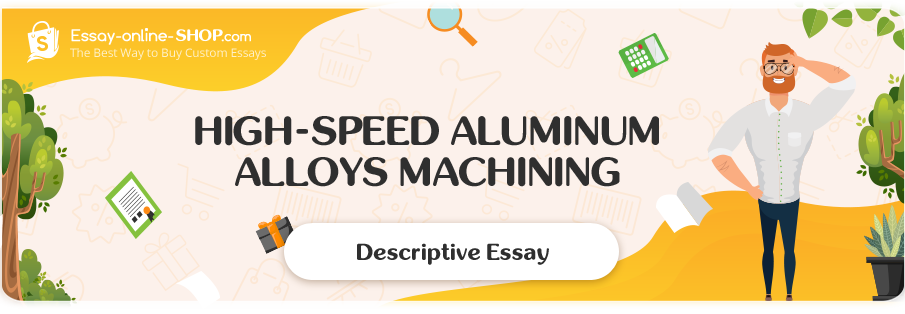
Abstract
Multiple uses of aluminum alloys in the manufacturing of various commodities cause the necessity for machining. It is used to shape the alloys for specific needs. Therefore, machining can be considered an important process in the preparation of aluminum. It is also an essential industrial practice. Like all similar operations, it has to be optimized to become economical and effective. This literature review prods scientific studies for information on the optimization of various factors that influence the effectiveness and costs of the machining process. They include alloy composition, the tool used, the force required, the depth and speed of the cutting tool, lubrication, reinforcement, and feed. An optimum combination of these factors will lead to the best machining outcomes regarding duration, final product quality, and cost involved. Every alloy is unique and, therefore, finding the specific combination of these parameters for the particular alloys is critical. The studies in this literature reviews provide different optimal parameters combinations for different alloys.
Keywords: aluminum alloys, machining, cutting force, cutting speed, reinforcement, lubrication.
The industrial use of aluminum alloys for manufacturing various products such as vehicles and planes has increased. Although it is possible to produce aluminum pieces exactly the way they are needed in the manufacturing process, machining is still necessary. It involves cutting, shipping, and smoothening of aluminum. The machining process depends on various factors. The results of several studies indicate that working on aluminum alloys is highly influenced by the composition of the alloy, the tool that is used, the force required, the depth and speed of the cutting tool, lubrication, reinforcement, and feed. Conversely, finding the optimal combination of all these factors is essential for working with alloys efficiently, producing perfect finishes, and ensuring economical use of the machining tools.

Factors Affecting Machinability of Aluminum Alloys
According to Santos et al. (2016), the machining of aluminum alloy has experienced expansion in the volumetric properties of the alloys. Now, the chip volume represents almost 80% of the initial amount in many areas of the industry (Santos et al. 2016, p. 3067). Based on the increased use of aluminum alloys, Santos et al. (2016) explored the machinability of the metal. Their review included various elements that influenced this indicator, such as machining conditions, cutting force, alloy composition, and cutting angle. The combination of these factors resulted in high-quality machining.
Jain, Soni and Baredar (2010) also explored various factors that affected the malleability of aluminum alloys. They provided machine parameters that must be considered when choosing aluminum alloys for machining. They include cutting speed, depth of cut, feed, tooling, and reinforcement, and their optimal combination is paramount for efficiency in the industry.
Lubrication and Roughness
Barzani et al. (2015) conducted research on the machinability of an aluminum alloy that contained 11% of silicon and 2% of copper (Barzani et al. 2015, p. 170). The study attempted to establish the impact of melt treatment in liaison with additional amounts of antimony and bismuth on the alloy’s machinability. Their findings revealed that workpieces that contained bismuth provided a better quality of surface roughness and required a lower cutting force. The part that contained antimony required a greater cutting force because it had greater surface roughness. In a nutshell, Barzani et al. (2015) suggested that bismuth could be used to improve the aluminum alloy’s machinability.
In their investigation, Najiha, Rahman and Kadirgam (2015) sought to determine the impact of minimum quantity lubrication optimization on the machining of aluminum alloys. The study strove to establish the need for lubrication for the efficient milling of the end product. The parameters used in the study were the speed of the cut, the depth, the feed rate, and the used tools. The findings of Najiha, Rahman and Kadirgam (2015) supported the need for lubrication to improve machinability. Similarly, the results of a study conducted by Puvanesan et al. (2014) indicated that lubrication of the machining tools led to high-quality finishes as it facilitates cooling and dissipation of the heat generated by the machining process.
Cutting
Cutting roughness and brittleness also determine the quality of machining. Barzani et al. (2015) demonstrated that low roughness required lower cutting force and resulted in better final products. Suparmaniam and Yusoff (2016) investigated the impacts of varying the combination of feed rate and cutting depth on the wear rate of the cutting tool using a metallurgical microscope. They also explored the surface roughness through a tester of the portable roughness of the surface the result after Aluminum and P20 steel milling. The findings of the investigation reveal that the surface roughness scores results for the influence of the feed rate although the impact was minimal.
The study by Wang and Liu (2016) on the strain rate on aluminum and different conditions during the speed cutting of aluminum alloy demonstrated that brittle fractures resulted in segmentation during the cutting process. According to Wang and Liu (2016), this tendency could be reduced through the use of larger strain rates. Moreover, larger applied force eventually affected the material removal during the machining process and impacted the quality of the final products.
The cutting force is essential in influencing various parameters in machining such as the duration of the process and the quality of the final product. As Zaghbani et al. (2011) observed the cutting force can also be influenced by the vibrations and their stability. In a study based upon these dynamics, Campatelli and Scippa (2012) analyzed the influence of the cutting speed and feed per tooth on cutting coefficients. They considered it a way to develop a consistent model with the ability to determine cutting forces accurately. The model they developed focused on tangential cutting coefficient for aluminum 6082-T4. Their finding indicated that the chip thickness and the cutting speed influenced the cutting ratio.
Salguero et al. (2013) also explored the impact of cutting force on the machining of aluminum alloys. According to Salguero et al. (2013), the material removal and cutting process were usually under the influence of various factors. They were the composition of the cutting tool as well as the geometry and nature of the workpiece. In their study, they analyzed how the cutting speed and feed rate influenced the cutting force during the high-speed milling of an aluminum-copper alloy. They approximated the force values for machining conditions and the variables subsequently proving that force heavily relied on the three variables.
- FREE revision (within 2 days)
- FREE title page
- FREE bibliography
- FREE outline (on request)
- FREE e-mail delivery
- FREE formatting
- Quality research and writing
- 24/7/365 Live support
- MA, BA, and PhD degree writers
- 100% Confidentiality
- No hidden charges
- No AI-written content
- Complete Authenticity
- 12 pt. Times New Roman
- Double-spaced/Single-spaced papers
- 1 inch margins
- Any citation style
- Up-to-date sources only
- Fully referenced papers
Thirumalai Kumaran et al. (2015) presented a better solution for the optimization of the cutting conditions during the machining of an aluminum alloy based on multiple elements of performance using gray rational analysis. Their study was conducted using Polycrystalline diamond tool to determine the best cutting condition with the aim of keeping surface roughness to the minimum level. Besides, they also tried to achieve reduced power consumption while at the same time maximizing the rate of material removal. To analyze the above objectives, Thirumalai Kumaran et al. (2015) took into account the feed, cutting speed, and cut depth. ANOVA (variance analysis) as well as the S/N (signal-to-nose) ratio were used in this study to establish the extent of the cutting parameters. The results indicated that the cutting speed had the greatest influence on the machining of the alloy (51.37%). The feed’s impact was second at 36.65%, while the effect of the cut depth stood at 11.66% (Thirumalai Kumaran et al. 2015, p. 17). The study subsequently optimized these parameters using confirmatory tests.
Some factors further influence cutting. In their study, Kalyan and Samuel (2015) investigated the effect of state of the art chamfer upon cutting approaches at feed rates in the high-speed machining of aluminum, magnesium, and silicon alloy through the use polycrystalline diamond tools. The approaches to cutting were established based on the force of the machining process, morphology, and roughness, at the varying rates of the chamfer age widths of the edge. Kalyan and Samuel (2015) demonstrated surface finish improvement alongside the increase in the radius of the tool in the shearing process. In the plowing process, larger radius deteriorated the surface finish. The two processes had different influences on the roughness of the edges.
The cutting process also depends on energy. Optimization of energy is paramount in the industrial setting. Wang et al. (2016) proposed a model for selecting parameters that could be used to reduce the amount of energy consumed by cutting during the machining of aluminum alloy 7050-T7451 (Wang et al. 2016, p. 292). The proposal that led to a cutting–edge reduction in power consumption was comprised of reduced friction during work as well as reduced deformation energy and chip kinetic energy. The study further revealed the optimal unreformed thickness of the chip, the cutting speed, as well as the tool rake angles for various cutting energy. However, due to the different behaviors of the aluminum alloys, they required different cutting forces. Therefore, researchers came to a conclusion that the optimization of the cutting force had to be specific for the product.
Wear Rate of Surface Material
A study by Suparmaniam and Yusoff (2016) tackled the impacts of varying the combination of feed rate and cutting depth to the wear rate of the cutting tool. The authors revealed that the wear rate of the device remained stagnant, an indication that the removal of materials had an enormous contribution to the tool’s wear rate. The absence of tool wear in their experiment was instrumental in proving that their HSM technique could be used in modern machining and would bring additional benefits such as increased productivity, semi-finishing operation elimination, and reduction of the tool load rate.
Different factors influence the wear. Shanmugavel et al. (2016) explored the optimization of dry sliding wear nature of mud-based aluminum compounds. The first parameters identified by Shanmugavel et al. (2016) included sliding velocity, applied load, the rate of reinforcement, and the toughness of the other material involved. Gray Rational Analysis was performed to achieve the optimization of the various elements involved simultaneously. The team used entropy approaches and principle component analysis to study the values of weights corresponding to the response of the output. Their findings indicated that the level of reinforcement at 34.9% was the most influential contributor to sliding wear filling by the velocity at 34.5% (Shanmugavel et al. 2016, p. 1).
Bardetsky, Attia, and Elbestawi (2005) also explored tool wear during high-speed machining of aluminum alloys. The presence of silicon in the alloy was attributed to a short tool life. Hard silicon components in the alloy increased tool wear. The tool’s fatigue wear occured in direct proportion to the percentage of silicon present in the alloy. Other influential factors in the tool wear included the grain size of the silicon and the loading condition of the tool. Bardetsky, Attia and Elbestawi (2005) developed a new model for the wear. Their concept embraced fracture mechanics and tangential and normal stress. The model proved effective at predicting the rate of wear using microstructure elements of the cutting tool, loading condition, and workpiece materials. Bardetsky, Attia and Elbestawi’s (2005) model was highly adaptable for cutting tool management in industries that use Al-Si alloys.
Similarly, Puvanesan et al. (2014) attempted to provide a mechanism for reducing the wear of the machining tool that could be very effective at reducing the costs of industrial machining. Through experimental investigations, they explored the impact of different quantities of lubricant and the characteristics of the milling tool after the machining process on aluminum alloys. The process parameters used in the study were cutting depth, speed, and rate. They were used to develop and optimize the base model. Puvanesan et al. (2014) found that the minimum quantity lubrication of the machining tool resulted in high quality and better finishes.
You can ask us “write my descriptive essay” on this or any other topic at Essay-Online-Shop.com. Don’t waste your time, order now!
Mechanical and Thermal Dispositions
The mechanical and thermal nature of the alloys also impacts their machinability. Davim et al. (2008) conducted a study on the mechanical and thermal changes that occurred during the machining of aluminum alloys. Their research used K10 (cemented carbide) and Polycrystalline diamond (PCD) tools and drew comparisons on various performance parameters between the tools. Davim et al. (2008) also used commercial finite element software which had the ability to report on various parameters such as temperature, cutting force, von Mises stress, pressures, plastic strain, and maximum shear stress. The study targeted all these objectives. Through analyses and simulations, Davim et al. (2008) found that compared to K10, the PCD tool was superior in performance-based feed and cutting forces as well as the temperature.
Okayasu et al. (2009) explored the properties of aluminum alloys from different machining approaches. Two methods were used in the study. They included very slow speed filling cold chamber and high-speed hot chamber die casting. A comparison between both methods revealed higher fatigue and mechanical strengths in hot chamber casting compared to the slow die casting. There were also remarkable differences in mechanical strengths, which influenced the machinability of the alloys.
Impact of Machining Approaches
The machining approaches are also influential in determining the roughness of an alloy. In a different study, Kuczmaszewski et al. (2016), analyzed the roughness of thin-walled aluminum alloy surfaces after milling. Their analysis focused on the roughness left by three different machining strategies as well as the rolling direction of the final product having the form of a plate in combination with the processed rolling feed. The research conducted by Kuczmaszewski et al. (2016) aimed at drawing a comparison between the different machining technologies and show which method was the best based on the surface roughness after the milling. Their findings recorded significant development in the aluminum machining processes.
Cast alloys are usually brittle, which at times makes it challenging to work with them. Kouam et al. (2013) evaluated the machinability of different aluminum alloys under different conditions. These include dry drilling alongside indexes, chip brittleness, form, and cutting forces. The alloys exhibited different behavior in various settings. The grain size of the chip also had varying influence under different machining conditions. It was found that material microstructure also affected the machining parameters.
Kumar Uthayakumar et al. (2014) experimented with the optimization of electrical discharge machining (EDM) elements of AL 6351 — an aluminum alloy that contains 5% of silicon and 10% of boron carbide (Kumar Uthayakumar et al. (2014,pp 1). Kumar et al. (2014) performed multi-response optimization using Grey Relational Analysis (GRA) to limit machining characteristics such as surface roughness, wear ratio of the electrode, and consumption of power. They identified the optimal input of the parameters that enhanced machinability using ANOVA (variance calculation). Their study revealed that pulse current caused more that 83% of the influence of the combination on the output responses (Kumar Uthayakumar et al. 2014, p. 1).
Machining techniques keep evolving, and new methods keep emerging (Benes 2008). However, as long as the factors that affect machining still exist, the final products’ quality will still be poor. Reducing the unnecessary factors that influence machining such as vibration is also important in improving the quality of the final products (Benes 2008). Younger and Eckelmeyer (2007) similarly indicated the different alloys had different characteristics and would, therefore, require different approaches to machining. The different requirement primarily include difference force for the cutting and tools among others.
Conclusion
High-speed machining of aluminum is essential for providing the metal or its alloys with a proper form for specified industrial uses. The process, however, can encounter many challenges concerning the machining process, chaining tools, the properties of the aluminum alloys, and the conditions under which the machining is done alongside other parameters. Various studies in the review above provide the different optimal conditions of the influencing factors and parameters for efficient machining of aluminum alloys.